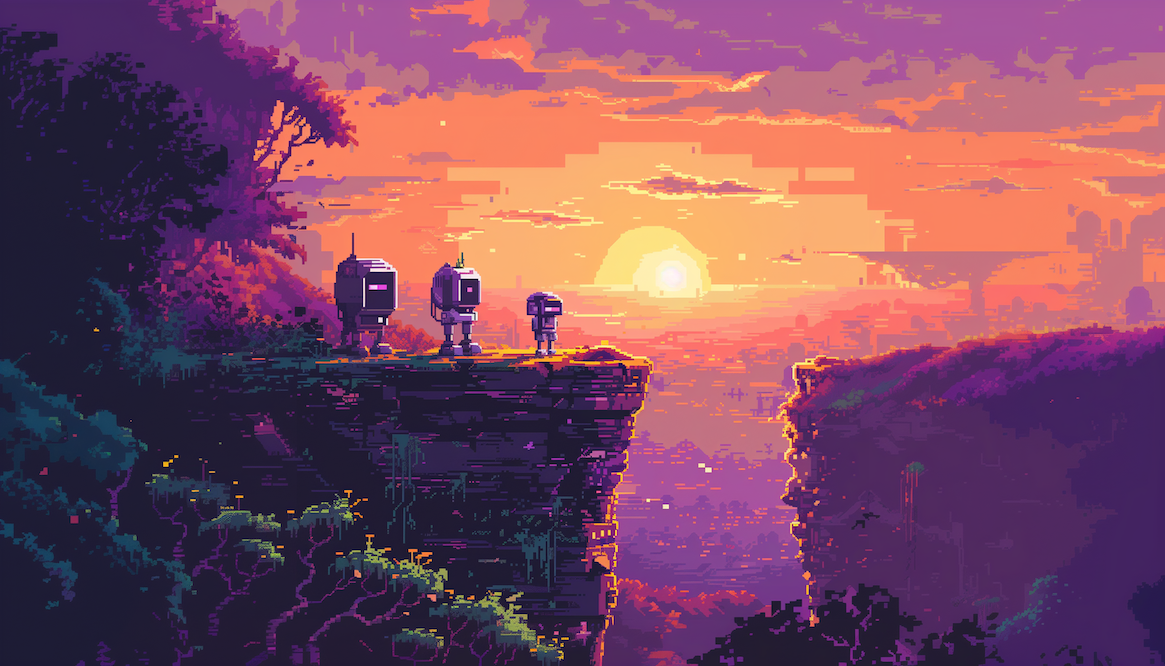
Sense, think, act. Robotics puts computation into motion. Imagine a future where AI isn't confined to the digital realm but moves and learns in our physical world. The next giant leap for AI isn't just digital; it's embodied.
In 2005, Linda Smith proposed the embodiment hypothesis, arguing that true human-level intelligence requires a physical presence, much like a baby learning by exploring its environment. While AI researchers remain divided on the thesis, we know that intelligence develops and learning compounds as we interact more and more with AI systems (i.e. Reinforced Learning from Human Feedback, or RLHF).
Until now, testing Smith’s thesis has been cost-prohibitive. AI needs a business model that affords incremental improvement, and robotics has always been less forgiving due to the associated hardware and data costs. A few years back, robotics research was very focused on reinforcement learning and overly specialized learning approaches, and it used a lot of data.
We're now seeing an emerging greenfield path for robotics: embedding LLMs into planning, VLMs into perception, and code generation into actuation. While it's all early, robotics will benefit from advances across the AI community—leveraging any improvements to language, multimodal models, video, and synthetic data generation.
These embodied systems will come in all shapes, sizes, levels of autonomy, and capabilities. Instead of plugging into a virtual world, we believe AI will populate our real environments with robots that can interact with and learn from our physical environments.
With these potential advancements in mind, we explore the changing market landscape, associated opportunities with robotic embodiments, and GTM strategies for robotics founders.
Why now?
Most people in the robotics industry know the phrase "robotics is hard." It's one of the nameless laws of nature that govern the field. Academically speaking, robotics embodies the Moravec paradox: what's easy for robots is hard for humans, and the inverse is true. But cheaper hardware, the onshoring of supply chains, and the adoption of standard protocols like ROS are enabling us to go from prototype to production faster than ever before.
We believe AI innovations will catalyze robotic application advancement across industries, and here are more reasons why we think there is suddenly a gateway for new competition:
- Robotics is multifactorial: Robotics is a systems problem—mechanical, electrical, sensing/perception, materials, firmware— fields each with their own development timelines, methodologies, and foreseeable issues. As the differentiation between software and hardware continues to blur, we expect it will be possible to scale development with smaller teams (e.g. "from physics and in simulation").
- Relying more on virtual simulators: The real world is unforgiving. Models train on examples taken from the real-world environment they represent. If conditions change (which they will) and the model doesn't reflect the shift, the model will decay and, eventually, fail. But, with new virtual simulations and deployment techniques like imitation-learning (learning from videos or humans to get a baseline model before you refine), teams can now further R&D before trialing in the cold, real world.
- VLMs empower a robot's contextual understanding: VLMs (visual language models) enable reasoning about visual observations (e.g. material, fragility, size) which could allow new robotic applications. Consider manufacturing, which requires robotic manipulation to understand and reason about the scene semantically and not just geometrically. For example, if a soft robotic gripper needs to handle fragile or irregular parts when building delicate chips across an assembly line
- Robots are great specialists but poor generalists: Typically, you have to train a model for each task, robot, and environment. Today, we can potentially generalize new capabilities by (1) correctly interpret a command (pre-trained language models breaking it into tasks), (2) visually identify all relevant objects within an environment (VLMs and real-time edge infrastructure) and finally (3) translate the instructions and perception into robotic actions (robotic foundation models)
- It all comes down to data: Data is hard to access, expensive, and of varying quality. What works within the confines of a lab, likely won’t translate to the lab next door and definitely not to the real world. We have early signs that collecting robotic data across diverse embodiments generates better performance than those trained on a single embodiment. Assuming this scales, this could be game-changing - Amazon is likely sitting on $100B worth of data licensing opportunities from their warehouses.
A map of the landscape
We believe robots will soon proliferate industries like logistics, defense, medical, pharma, agriculture, construction, mining, and more. Whenever you’re at the beginning of an arms race, it's good to be an arms dealer. But what do the new arms dealers for robotics sell? Historically, we only have a few examples of large robotics-enabling companies - Applied Intuition (autonomy software, $6B valuation), Scale AI (data labeling, $13B valuation), and Seyond (LiDar, $1.2B valuation).
Some of these technologies will likely be integrated into other infrastructure platforms as they shift to capture the robotics markets; others will just be built by internal teams, while others could become standalone businesses:

How robotics is taking new forms
We’re seeing some trends and opportunities across robotic form factors:
(1) "Fenced Offs": Large industrial robots, such as Kuka arms, require secure installations and physical barriers for human safety. Although these systems are powerful and equipped with sophisticated control systems, they are primarily valuable in high-volume applications like pick and packing. With advancements in safety, sensing, and controls, we are gradually moving towards more flexible, fence-less, and collaborative configurations.
(2) Humanoids: Companies from Figure and Boston Dynamics to Agility and Apptronik are pushing the envelope with robots that resemble humans. Although humanoid robots offer visually impressive demos on Twitter, creating robust controllers and balancing systems is highly challenging, and most production environments do not necessitate a humanoid design.
(3) Cobots: Collaborative robots are designed to work side-by-side with humans, and we expect this to be the dominant trend in the next few years. The challenge lies in crafting interactions and behaviors between humans and machines that foster trust. While social robots have been around for decades, historically, functionality was prioritized over effective human-robot interaction. Disney's approach to robotics demonstrates the potential for robots to communicate through character without compromising their functional roles.
(4) Tiny AI: We're shifting towards specialized, small devices, each designed for specific tasks. These devices offer targeted functionality, affordability, and enhanced privacy since they don't rely on cloud infrastructure. This design eliminates the need for subscriptions and personal data collection and allows for independent operation and easy upgrades. Soon, our spaces will seamlessly integrate with these intelligent, modular objects that assist us without intruding, functioning independently, and requiring minimal configuration.
Building for tomorrow: The components of a robotic team
We’ve respected the approach and company-building strategies of Cobots, Hadrian, Sheep Robotics, Machina and Monumental Labs. As investors in the first wave of vertical robotics — Farmwise with agricultural robots, Zipline with drone delivery, and Avidbots for cleaning — we’ve spent a lot of time thinking about what will separate outlier robotics teams in the future. We’ve given this framework the acronym STACKED, because great teams understand how to stack the deck in their favor, which is necessary given the complex industry that is robotics.
- Specify the Problem: Don't fall victim to the curse of generality. A system designed to do everything is unlikely to do all equally well, which is bound to create disappointment. Remember that many companies use robotics to enhance their operational margins.
- Tackle software first, not hardware: Focus on understanding what robots are capable of today, deploy quickly and leverage machine learning and AI advances to improve further and streamline operations.
- Approach software for your growth model: Traditional robotics is all about robotics as a service (RAAS), but with AI—this approach fails to capture the market potential. Integrating AI, software, services, and robotics can be incredibly powerful across vertical, horizontal, or diagonal business models. Suppose you're deploying robots within your own business: your margins will improve alongside your model's development (e.g., improving collecting, processing, and data quality) and can afford you more bandwidth to absorb the costs of mistakes and years of required refinement.
- Choose your data profitability breakthrough: Building a data engine that remains cost-efficient at scale (i.e., your data profitability breakthrough) is difficult and expensive. Robotics is edge-constrained, so success is often dependent upon data flywheel loops and continuous ingestion of this fresh data to keep pace with your bar for performance; it's about finding a balance between A) constraining the problem enough to get to the right level of automation/scalability; B) hiring "humans-in-the-loop" to cover edge cases where models don't perform well, and; C) still finding a good enough value proposition and a large enough market.
- Know your weaknesses: Don't build everything. With the standardization of hardware and protocols and growing off-the-shelf infrastructure, a robotics team should be able to ship faster. Instead, focus on creating a data flywheel for a model to mature and gradually provide business value without meaningfully increasing capital expenditures (CapEx).
- Embodiment design must reflect function: A robot's physical form dictates everything from processing power and battery life to communication capabilities and sensory awareness. It also sets expectations about its performance. It's essential to deliver or over-deliver on its promise, or it will not be embraced. Choose designs that enhance rather than diminish user interaction, especially in environments shared with humans.
- Deploy, deploy, deploy: Launching simple, practical solutions quickly and refining them through real-world experience is critical. This approach allows companies to learn, adapt, and improve without waiting for perfect solutions. Early deployments can provide valuable insights that guide more significant investments and technological advancements.
Embodying intelligence moving AI forward
While much excitement surrounds the impact of AI on our lives, to date, the conversation has focused primarily on software. This overlooks the substantial opportunity for integrating AI into robotics and the role these smart machines can play in enhancing our lives and the field of AI.
With exciting approaches utilizing advancements in LLMs, VLMs, and code generation, robotics makers have a tremendous opportunity to develop more innovative machines at scale. Meanwhile, as market shifts remove limitations around robot R&D, it will afford founders more financial leeway to utilize AI in robots effectively.
It's clear that embodiment will be a vital tool in reshaping our physical world in the coming years and we are excited to collaborate with teams who also dream this dream. If you are building something at the intersection of robotics, hardware, and AI, we'd love to connect! Reach out to us at robotics@felicis.com